Investment Centre
Using AI to help in determining if past performance is a predictor of future success
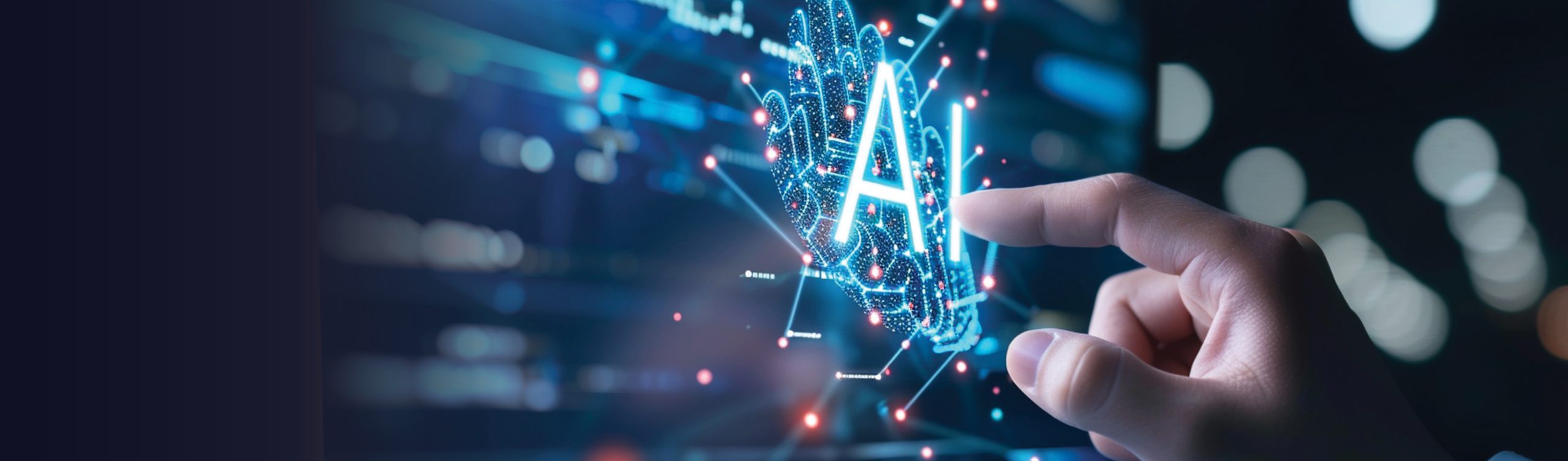
The test
Investors often study historical fund performance relative to industry peers when selecting an investment fund/portfolio, and from experience, a 3-year performance number is a popular reference point.
But how useful can short-term performance really be? Or is this a flawed approach?
In this article, using past performance, we analysed the ASISA Multi-Asset High Equity category. This category is the least restrictive among the Regulation 28 compliant categories, allowing asset managers ample scope to demonstrate multi-asset class skill. The category also contains well-known balanced funds managed by Allan Gray, Coronation and Ninety One.
We extracted four sets of three-year non-overlapping (this is very important) annualised returns from July 2011 to June 2014 (period 1), July 2014 to June 2017 (period 2), July 2017 to June 2020 (period 3), and July 2020 to June 2023 (period 4). For a fair comparison, only retail fee classes that had returns over a full period, were included. We tested how funds with three-year returns, in any two consecutive periods, performed from one period to the next. To speed up the exercise, we employed the help of artificial intelligence, using ChatGPT Plus and its Code Interpreter. We provided ChatGPT with all the fund returns and through multiple prompts, generated the analysis.
The process required trial and error, but we were highly impressed by the functionality. The outcome of the analysis was separately verified.
Results: does top quartile performance persist?
Based on a small sample size of 15 top quartile performers in period 1, five funds remained in the top quartile over the next three years – slightly better than the four funds (25% of the 15 funds rounded up) expected if relative performance was random i.e., if funds had an equal chance of appearing in each of the four quartiles. These funds on average outperformed by 0.6% p.a. in the subsequent period.
In period 2, of the 22 funds that were top quartile performers (note an increase in the number of funds as new funds were launched over time), seven funds remained in the top quartile in period 3, again slightly above what would have been expected if relative performance was random. However, on average these 22 funds underperformed the peer group average by 0.2% p.a. in the subsequent period.
When testing period 3, the sample size of funds was 157 – almost double that of period 2. Amongst the 39 top quartile funds, only four of these funds remained top quartile over the next three years. This is significantly below the 10 funds expected if relative performance was random. On average these funds underperformed by 1.5% p.a. The table that follows summarises the findings
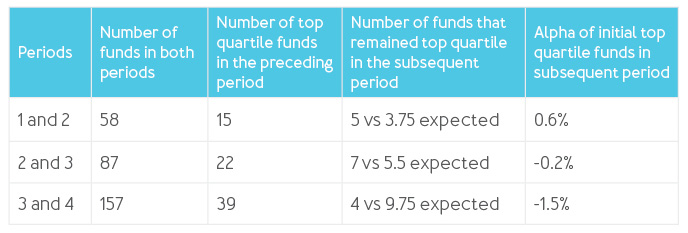
Therefore, as illustrated in the table, investing equally in the top quartile funds over the past three years is no guarantee of future outperformance, and could even lead to material underperformance.
Results: regression analysis insights
Using an alternative approach, we also asked ChatGPT Plus to perform a linear regression to establish if alpha over the initial three years (explanatory variable) had any predictive power of alpha over the subsequent three years. The advantage of this analysis is that it incorporates data from all the funds per period, not just top quartile funds, thus increasing the information available.
To further increase the power of the test, we combined the data from all the periods used for this exercise. Over the periods tested, the results suggest that past quartile performance and alpha, provide no insights into future quartile performance and alpha. It will also be interesting to expand the analysis to more ASISA Multi-Asset categories to determine if the results hold across multiple categories.
A strong indicator of this weak relationship is the regression’s low R-squared value of 1% (R-squared indicates how much of the variability of the data is explained by the regression). A result of 1% is very little, i.e., the model only explains 1% of the relationship between the x and y variables.
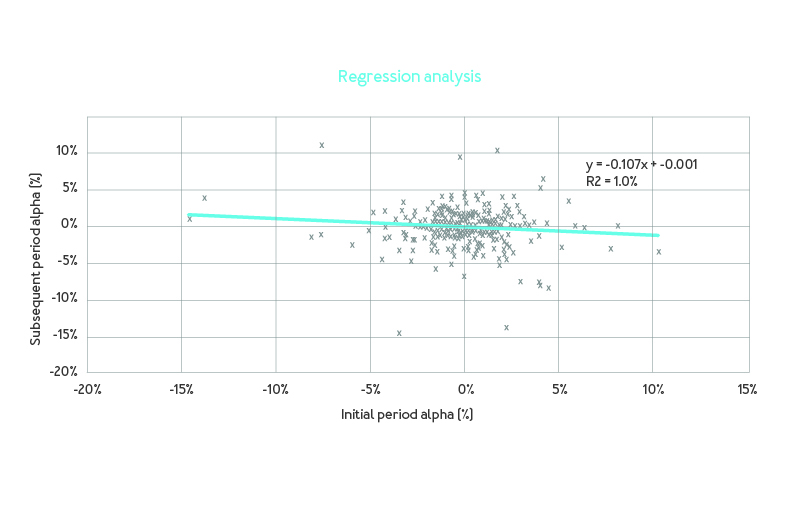
Furthermore, for the more numerical investor, in determining the significance of the regression, the p-value of the slope of the regression line is helpful. The p-value is the probability of observing these results under the null hypothesis that there is no
linear relationship. It is approximately 8%, which is only slightly above the common significance threshold of 5%. This means that the relationship between the initial period alpha and subsequent period alpha is not statistically significant, at the 5% level of significance.
If, however, you wanted to conclude that there was a relationship at a slightly lower level of significance, it would in fact be that outperformance in one period, is more likely to lead to underperformance over the next period i.e., you should be buying
the underperforming funds. We would caution against that relationship and rather conclude that there is no relationship between past and future relative performance – little variability is explained by the relationship i.e., statistical significance would
not imply economic significance in this case.
How then to select a future outperforming fund
It was interesting to test how ChatGPT Plus can help improve analytics. We are increasingly testing such AI tools to see how they can aid analysis and decision making, and we can certainly see the use of this technology growing exponentially.
If ChatGPT tells us that fund alpha over the past three years provides limited insights into future alpha, how does one construct an outperforming solution for investors?
It is important to understand that a three-year track record provides limited insights, with an array of factors affecting outcomes, making the identification of asset manager skill very difficult. Therefore, our approach is a combination of in-depth qualitative and quantitative analysis with an operational due diligence overlay. Our quantitative analysis is also not only performance-based but includes numerous performance and risk-based measures. Understanding performance in the context of a fund’s investment strategy, style, and positioning, is a key objective for us and our team spends thousands of hours on this each year.
Our approach is forward-looking – trying to understand the past to help us with future expectations. Similar to asset managers that diversify portfolios by including a number of shares, we diversify across multiple managers and strategies that we think have the potential to outperform in the years to come. Our process stacks the odds in our favour.
In our opinion, this forward-looking approach is a far superior approach compared to merely selecting from the latest performance ranking tables. This is evident in our consistent rankings in either the first or second quartiles over various time periods.
Key Points
- A three-year track record, investing equally in the top quartile funds is no guarantee of similar success over the next three years.
- Linear regression analysis indicates performance over the past three years is no indication of performance over the next 3 years.
- A combination of in-depth qualitative and quantitative analysis is a better strategy to build outperforming solutions.